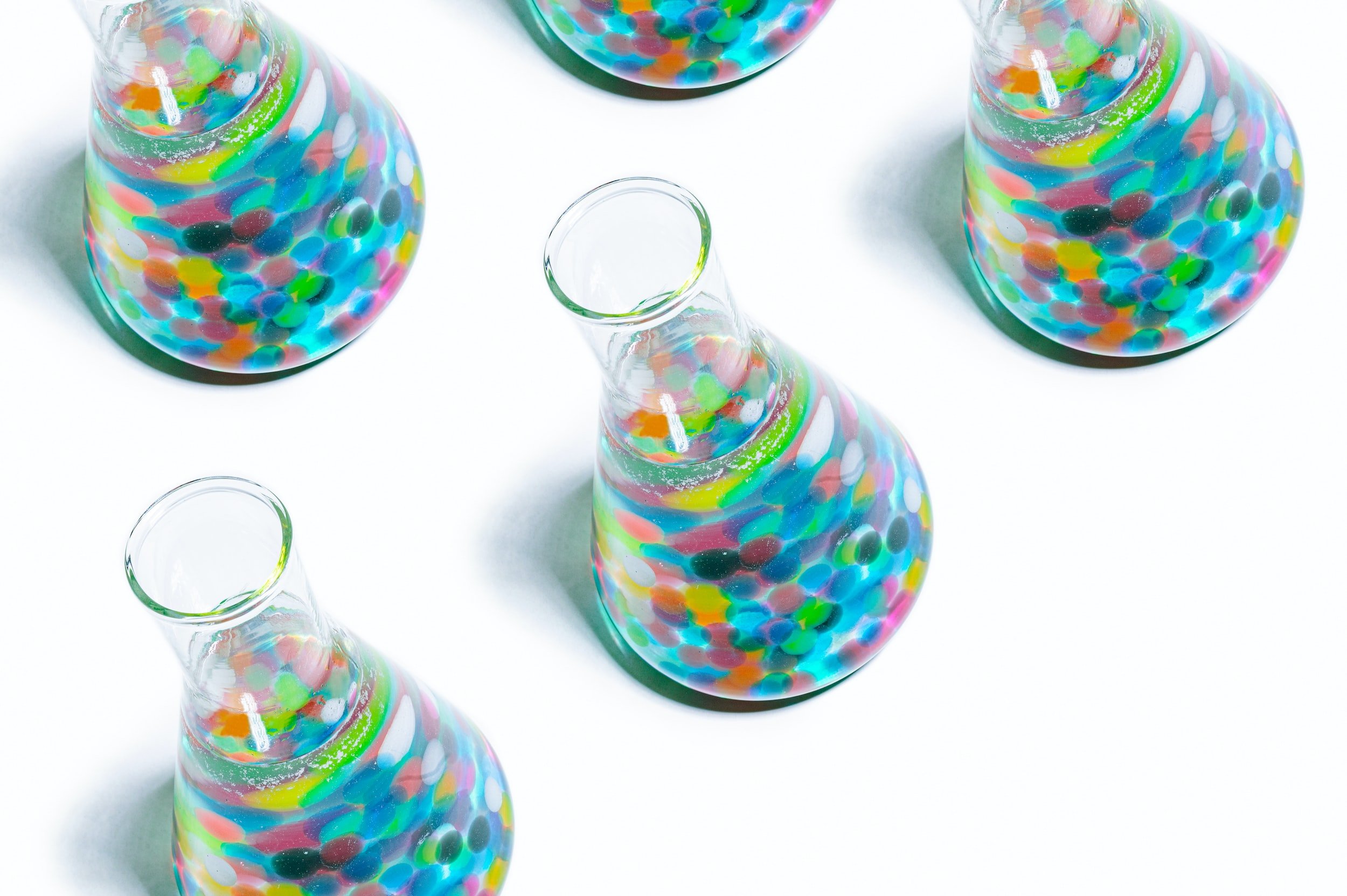
Insights & Perspectives for the Biopharma, Biotech, Academic and Financial Communities
,
SugarCone Biotech evaluates, consults and executes novel platforms and therapeutics across multiple disease areas including oncology, immuno-oncology, cell and gene therapies, inflammation, autoimmunity, and fibrosis.
Blogs, News, & Whitepapers
Featured Insights
what we do
About SugarCone Biotech
Our role at SugarCone Biotech is to remain fiercely up-to-date on the core discovery science of immunity, the translational and clinical sciences that are impacted by novel therapies, the treatment landscapes of diverse indications and the competition across diverse disease areas.